Global Site
Displaying present location in the site.
Disaster Damage Assessment Using LLMs and Image Analysis
Vol.17 No.2 June 2024 Special Issue on Revolutionizing Business Practices with Generative AI — Advancing the Societal Adoption of AI with the Support of Generative AI TechnologiesAgainst the backdrop of the intensification of torrential rain disasters in recent years and concerns about the occurrence of a massive earthquake in the near future, there is a growing demand for further strengthening disaster control measures. In the event of a disaster, it is crucial to assess disaster damage quickly and accurately to minimize damage by expediting initial response activities such as evacuation guidance and rescue efforts. In this paper, we introduce a technology that utilizes a multitude of field images collected in the event of a disaster to assess the damage through the use of large language models (LLMs) and image analysis.
1. Introduction
In recent years, many regions around the world have faced an increase in the frequency and severity of natural disasters, including intensified torrential rains and massive earthquakes. In times of disaster, it is crucial to quickly and accurately assess the damage to expedite initial response activities such as evacuation guidance and rescue efforts for disaster victims. However, because precipitation distribution maps, seismic intensity distribution maps, and other textual information provided by relevant government agencies as well as reports from residents about damage and safety during disasters may lack detailed information about the disaster damage and location, challenges still exist in the realization of a rapid initial response. In contrast, images from disaster-stricken areas provided to municipalities (images captured by smartphones, drive recorders, CCTV, etc.) contain extensive information about the disaster damage and geographical location and hold great promise.
In this paper, we introduce a technology for disaster assessment that quickly and accurately finds the images necessary for assessing the disaster damage from a multitude of field images and displays them on a map with street address-level accuracy, aiming to expedite initial activities.
2. Features
The technology for disaster assessment enables users to search for and display the necessary images from a multitude of field images on a map (Fig. 1). In section 2, we will explain the features of the AI for narrowing down images and location estimation that make this possible.
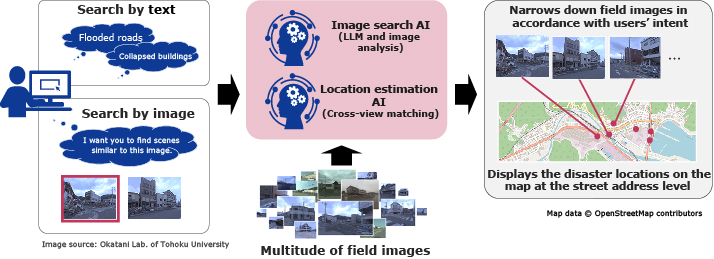
2.1 AI to narrow down images in search results
When searching for images, images can be narrowed down from a multitude of field images to include only those that align with the user’s intent by utilizing the semantic interpretation of words with a large language model (LLM) and the similarity judgment of images with image analysis.
Image recognition has conventionally been used for narrowing down images to those that are relevant, but it was only capable of recognizing specific pre-learned objects, thereby limiting which images can be narrowed down in a search. This makes it challenging to conduct accurate surveys in accordance with a user’s intent, which varies depending on the type and scale of a disaster, the affected areas, and the extent of the situation.
This technology utilizes LLMs uses keywords input by the user to narrow down field images. Furthermore, using image analysis and specifying scenes the users would want to search for makes it possible to narrow down the search to similar images that are difficult to express in words. By combining LLM with its textual prompts and image analysis in this way, users can accurately narrow down the images to only those matching their intentions, making it possible to quickly respond to changing situations of disasters as they unfold.
2.2 AI to estimate the location of damage
In location estimation for field images where the disaster location is unknown, the location of the field image can be estimated with street address-level accuracy and then displayed on a map by comparing (cross-view matching) the images with aerial imagery and map data covering extensive areas.
Field images provided in emergency situations such as disasters do not necessarily include location information, making it sometimes difficult to identify disaster-stricken areas. While NEC has previously developed technologies for estimating locations through the use of aerial images such as satellite images and aerial photographs1), this latest technology has achieved the world’s highest matching accuracy2) by leveraging geographic information from map data. This enables location estimation with a high degree of precision, even for field images captured during disasters.
This technology automatically extracts areas such as roads, buildings, and traffic signals from field images and then estimates locations by matching them with map layout information (the shape and layout of roads, buildings, etc.). In this way, information on roads showing a lower risk of damage is proactively used in the event of an earthquake, and during floods, information on buildings showing a lower risk of submergence in the event of flooding is used to perform matching. This enables highly accurate estimation of a photographed location, even for field images of partially collapsed buildings or partially flooded roads.
3. Application
Fig. 2 shows an application that uses this technology for assessing disaster damage from a multitude of field images. Through interactive communication between the user and the system, the images are narrowed down (left), and the locations of the resulting images are displayed on the map (right). In the example shown in Fig. 2, a search is conducted to find images of collapsed buildings, using textual prompts. Then by selecting and specifying images with significant damage from the search results, it is possible to narrow down the number of scenes that are difficult to express in words, such as the extent of the damage. The resulting images are displayed on the map based on the information obtained from location estimation. In this way, by using this technology, users can quickly assess a variety of disaster situations they want to investigate.
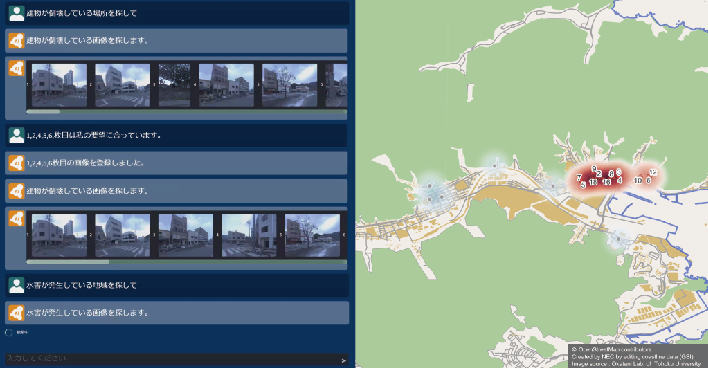
4. Conclusion
In this paper, we introduced a technology that utilizes a multitude of images from disaster sites to swiftly and accurately assess the situation using LLMs and image analysis. NEC plans to further develop this technology for practical use in an effort to help accelerate evacuation guidance, rescue efforts, and other initial response activities in the event of a disaster. In addition, NEC remains committed to making society safer, more secure, and more convenient by expanding the use of LLM and image analysis technology to other applications.
References
- 1)
- 2)
Authors’ Profiles
Director
Visual Intelligence Research Laboratories
Director
Visual Intelligence Research Laboratories
Researcher
Visual Intelligence Research Laboratories
Senior professional
Visual Intelligence Research Laboratories
Professional
Visual Intelligence Research Laboratories
Senior researcher
NEC Laboratories Singapore