Global Site
Displaying present location in the site.
Patient Condition Change Signs Detection Technology for Early Hospital Discharge Support
The growth in hospitalization periods is raising concern with regard to increased social security expenses. Delays in hospital discharge of patients are mainly caused by two issues. These are the extra treatment required due to changes in the condition of inpatients and also by the delay of hospital discharge scheduling due to inefficient office procedures. This paper introduces efforts being made regarding agitation and aspiration pneumonia issues. These are the main factors causing delays due to the need for extra treatments. NEC Corporation has developed an AI technology that detects agitation and aspiration pneumonia based on the learning of electronic medical records and vital signs. The use of AI technology in this topic, which has been considered as being difficult to detect before it actually occurs, enables advance intervention by medical personnel and also supports the prospect of earlier patient discharge.
1. Introduction
The increase in social security costs due to the aging population is an important issue for Japan. The Ministry of Health, Labour and Welfare is tackling the hospitalization charges as a measure for optimizing the social security expenses. One of the means for hospitalization charge optimization is to avoid delays in the hospital discharge of patients and one of the factors causing the hospital discharge delays is the onset of complications during hospitalization. A typical example of a disease that extends the hospitalization period is cerebral stroke and it has been reported that agitation and aspiration pneumonia are the complications occurring most often among the stroke patients. Past electronic medical record data also shows that delays caused by agitation extends hospitalization by about 19 days and delays caused by aspiration pneumonia extends it by about 14 days compared to the average hospitalization period. (Data of the Kitahara International Hospital being ranked the 4th in the neurosurgical treatment achievements in Tokyo in 2019). NEC has conducted joint research with the Kitahara Group, aiming at avoiding complications by detecting the signs of patient condition changes that would lead to the prediction of agitation or aspiration pneumonia, so that proper measures can be taken.
In order to detect agitation and aspiration pneumonia, conditions that have previously been difficult to detect in advance, NEC has developed an AI technology that performs learning based on electronic medical records and vital signs. This AI technology makes possible advance intervention by medical personnel and also presents the prospect of earlier patient discharge. The rest of this paper will introduce details of this technology.
2. Detection of Agitation Signs
2.1 Agitation sign detection technology
This section describes the agitation sign detection technology that forms a part of the condition change signs detection technology.
Agitation refers to disorders (hallucination, delusion, emotional instability, disarray) that tend to occur among inpatients accompanying delirium, etc. When agitation occurs in a patient hospitalized for an acute disease, the risks of treatment delays due to self-removal of drip infusion or oxygen cannula and injuries caused by falling from the bed or tripping over while walking will increase as shown in Fig. 1.
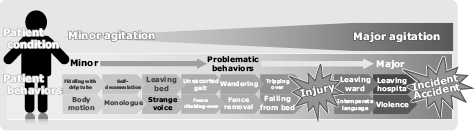
The analyses of medical records at the Kitahara International Hospital have shown that agitation is observed among about 34% of inpatients. The discharges of patients with agitation were delayed by about 19 days compared to other patients. A verification conducted with a small amount of offline data acquired at the Kitahara International Hospital (data of about 140 hours of 9 inpatients) showed that the changes of the heartbeats and body temperatures with time were correlated with the agitation. As shown in Fig. 2, the time-series data of the heartbeats (peak intervals of the photoplethysmographic waveform) and skin temperature are collected as the explanatory variables. Subsequently, the agitation identification model is generated based on the feature quantities calculated from the time-series data mentioned above. The agitation data that is the possible and negative instance data is generated from the video data. The agitation is predicted according to the time-series data of heartbeats and skin temperature as well as from the agitation Identification model (Fig. 3).

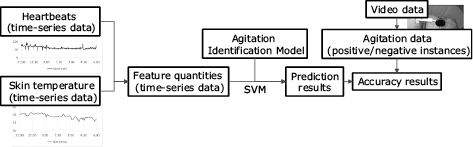
2.2 Evaluation
The agitation sign detection technology was evaluated at the Kitahara International Hospital by building a large-scale data collection experiment environment shown in Fig. 4 inside the hospital.
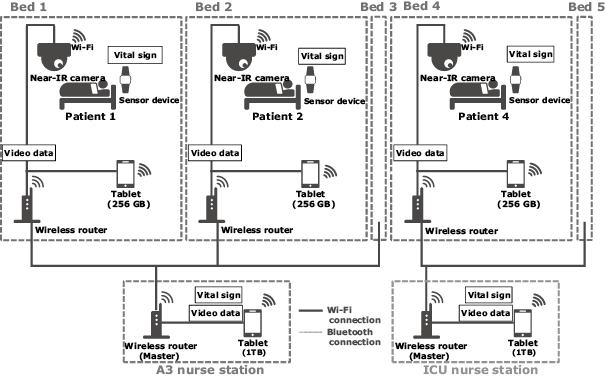
Data from a total of 318 nights was collected from July 2018 to February 2019. The data was cleansed and divided into three for evaluation. The evaluation results are shown in Table 1. The advance detection rate of agitation was 78%. Although the detection error rate was about 1/4, it has been confirmed by hearing the opinions of nurses that for them this result was acceptable.
Table 1 Agitation prediction results
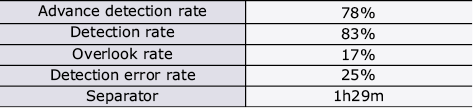
As the results above showed that the agitation signs detection in the neurosurgical department can offer a detection accuracy acceptable by medical personnel, a real-time agitation sign detection demonstration experiment environment was developed. In this environment, when the vital signs (heartbeats, etc.) of a patient are acquired, the patient data is saved for analysis at a terminal. If the patient data is identified to present a risk of agitation, an alert is displayed on the terminal.
In future, the value indices of detecting agitation signs in real time and alerting the medical personnel (the effects of reducing the burden on medical personnel, reduction of the risk of incidents with patients, and of enabling early discharges) will be evaluated.
3. Prediction of Aspiration Pneumonia Risks
3.1 Aspiration pneumonia risk prediction technology
This section describes the aspiration pneumonia risk prediction technology that is a part of the condition change signs detection technology.
As described above, aspiration pneumonia most often occurs as a complication of brain stroke patients. Particularly with aged persons, the degradation of the swallowing function increases the risk further1). An aspiration pneumonia risk prediction model was compiled from 8,000 items of patient data obtained from inpatients in the past five years at the Kitahara International Hospital. The data used in the prediction model is based on information including patient condition and the positive and negative instance data are classified by whether or not the antibiotics used for treatment of aspiration pneumonia at the Kitahara International Hospital is administered. One of the issues in compilation of the prediction model was the tendency to overlook caused by non-uniformity of the data, so a training technique for reducing overlooking was introduced2). Fig. 5 depicts the learners in the prediction model.
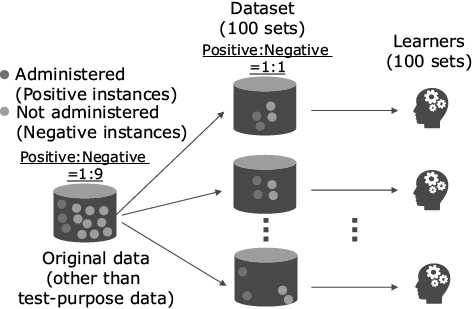
To evaluate the prediction model, the algorithms of the learners were compared. The performance evaluation results of the prediction model were as shown in Table 2, which indicates that the prediction model prototyped for the present experiment achieves a higher sensitivity as well as AUC (Area Under the Curve) compared to the general model (logistic regression) while their specificities are identical. It is thought that, to prevent overlooking the presence of aspiration pneumonia, high sensitivity and AUC are important when the specificity is the same. Therefore, it was decided to use the prototyped prediction model in the demonstration experiment.
Table 2 Performance evaluation of prediction model
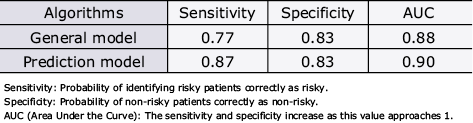
3.2 Aspiration pneumonia risk prediction
The aspiration pneumonia risk prediction demonstration experiment at the Kitahara International Hospital was conducted over a three month period from August to October 2018. The outline of this demonstration experiment is shown in Fig. 6. The AI technology predicting the risk outputs the aspiration pneumonia risk prediction results in the early period after hospitalization (the fourth day after hospitalization in the present experiment). The medical staff then applied the preventive care for one week according to the output results. The preventive care includes the bed angle increase, breathing and abdominal pressure exercises and the evaluated item was the number of patients showing aspiratory pneumonia within one week starting from the fifth day after hospitalization.
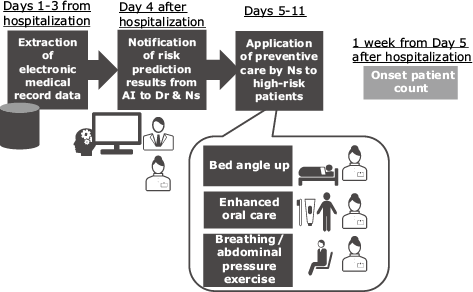
Table 3 shows the comparison of patients showing aspiration pneumonia in the week starting from the fifth day after hospitalization between the times before and after the demonstration experiment. The number of aspiration pneumonia patients in the three months before the demonstration experiment was seven while those within the experiment period numbered 0. Although the effects were confirmed in the demonstration experiment, NEC still considers that the number of subjects was insufficient from a statistical viewpoint. In the future, the number of test subjects will be increased in order to improve the evaluation accuracy. It is also planned to verify the effects of early hospital discharges.
Table 3 Comparison of patients presenting aspiration pneumonia
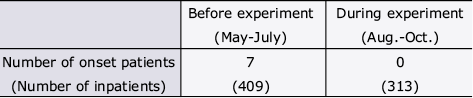
4. Conclusion
In the above, the authors introduced the AI technology that performs learning based on electronic medical records and vital signs in order to detect agitation and aspiration pneumonia, conditions that have previously been difficult to predict before they occur. The authors have the prospect that the use of this AI technology will enable intervention by medical personnel before the onset of issues and that this would lead to early hospital discharge. In the future, it is planned to advance demonstration experiments aiming at improvements in prediction accuracy and real-time detection.
- *Wi-Fi is a registered trademark of Wi-Fi Alliance.
- *Bluetooth is a trademark owned by the Bluetooth SIG, Inc., and is used by NEC Corporation under license.
- *All other company and product names mentioned are trademarks and/or registered trademarks of their respective owners.
References
- 1) Shinji Teramoto, Yoshinosuke Fukuchi, Hidetada Sasaki, Koichi Sato, Kiyohisa Sekizawa, and Takeshi Matsuse: High incidence of aspiration pneumonia in community- and hospital-acquired pneumonia in hospitalized patients: a multicenter, prospective study in Japan, Journal of the American Geriatrics Society, vol.56, Issue 3, pp.577-579, February 2008
- 2) Leo Breiman: Bagging predictors, Machine Learning, vol.24, Issue .2, pp.123-140, August 1996
Authors’ Profiles
Senior Researcher
Data Science Research Laboratories
Senior Researcher
Data Science Research Laboratories
Principal Researcher
Data Science Research Laboratories