Global Site
Displaying present location in the site.
Understanding of Behaviors in Real World through Video Analysis and Generative AI
Vol.17 No.2 June 2024 Special Issue on Revolutionizing Business Practices with Generative AI — Advancing the Societal Adoption of AI with the Support of Generative AI TechnologiesNEC believes that it is important to understand behaviors in the real world to achieve safety, security, fairness, and efficiency. However, it is difficult to understand complex or unexpected behaviors with conventional video analysis. Therefore, we propose the use of a technology that understands real-world behaviors and their context and that also predicts the intentions behind the behaviors as well as future actions by utilizing the latest generative AI. In this paper, we propose specific architectures that can achieve an understanding of real-world behaviors by using video analysis and generative AI, and we present the results of an experiment that demonstrated it is possible to understand suspected behaviors in office buildings with the proposed architecture.
1. Importance of Understanding Real-World Behaviors
NEC aims to achieve a sustainable society where social values of safety, security, fairness, and efficiency are created and where anyone can fully demonstrate their humanity. Specifically, we are focusing on crime prevention, improved public safety, elimination of danger and congestion in cities, and monitoring of the elderly and children. To effectively promote these initiatives for people’s safety and security, a deep understanding of how people behave in the real world is critical.
2. Challenges in Conventional Video Analysis
To understand the behaviors of people in the real world, NEC uses video analysis technologies including face recognition, entry/exit/stay control, and behavior detection to provide a variety of solutions such as face identification and human attribute analysis using camera images, marketing by people-flow analysis, and safety management at work sites.
However, the current video analysis technology is unable to sufficiently understand complex behaviors—especially unexpected behaviors—of people in the diverse and drastically changing real world because it applies a preconceived model to recognize simple actions and behaviors. In addition, the challenge is that it is difficult to understand the intention of behaviors or to predict behaviors merely by recognizing simple actions and behaviors.
3. Use of Generative AI and Its Effects
Large language models (LLMs), which have developed significantly in recent years, can understand the complex context of texts written in natural language and generate appropriate response texts. LLMs are about to evolve into large generative AI models (LGAIMs) that can understand and generate not only texts but also still images, moving images, point clouds, audio, structured data, chronological data, and other formats.
NEC believes that current challenges in video analysis can be solved by utilizing LLMs and LGAIMs to understand behaviors in the real world. This is because these technologies can understand the context of people’s behaviors from images and other information taken from the real world, leading it to understand the reasons and intentions behind the behaviors and to predict what the people will do next. And then, by understanding the reasons and intentions behind a behavior, we can provide high-value services that support behaviors or prevent risks by predicting the next behaviors (Fig. 1).
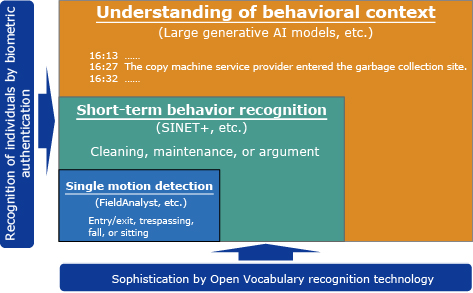
4. Architecture of Understanding Behaviors in the Real World
4.1 Recognition and recording of events in the real world
To achieve an understanding of behaviors in the real world, you first need to recognize and record individual real-world events. We expect that LGAIMs will be able to recognize individual events in the future, but conventional video analysis should be utilized at present.
Also, to record people’s individual behaviors as a series of behavior records, biometric technology to identify individuals and identification technology with information from multiple cameras should be utilized. Identification of individuals could be done in places like factories and offices where the individuals being photographed or filmed can recognize that their personal information is being obtained and for appropriate purposes. If said conditions cannot be achieved, identification technology that does not identify individuals but instead is based on clothing and other characteristics in appearance should be utilized to ensure anonymity.
In addition to video analysis, audio recognition or acoustic analysis can be utilized to recognize individual events in more detail.
Events recognized in this way are recorded in the database as structural data and include individual identifiers that indicate who, when, where, and what as well as the date, time, location, and actions/behaviors (Table).
Table Example of behavioral event data.
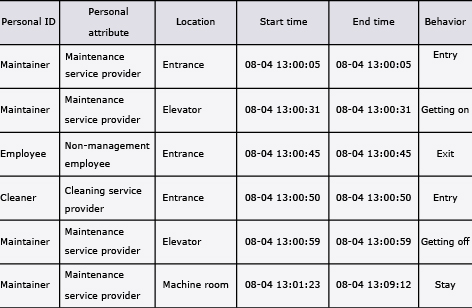
Furthermore, the feature vector (embedding) representing the semantic features of actions and behaviors will be extracted from texts, videos, etc. and recorded.
4.2 Understanding of behaviors by generative AI
The events that occurred for each individual during a particular period are extracted from a database in which events in the real world have been recorded as previously described.
Next, text records of the extracted events are arranged in chronological order and presented to the LLM for analysis by submitting the records together with instructions such as “Check the behavior records for any suspicious behaviors.” Generally, the number of tokens that can be processed by generative AI is limited, so it is impossible to input all the events that occurred over a long period. For this reason, NEC makes it possible for the LLM to analyze the events that occurred over a long period by extracting only the events necessary for the analysis or by summarizing the events. Also, by describing events with general terms in verbalizations, it is possible to make analyses utilizing knowledge from the general world even in a highly unique real-world environment such as a plant.
In an analysis in a highly unique real-world setting, the accuracy of generative AI analyses can be improved by in-context learning if other general behavioral events or other information with similar feature vectors are extracted and added as reference information. When LGAIMs can be utilized, higher-precision analyses will become possible by inputting video and audio of events (Fig. 2).
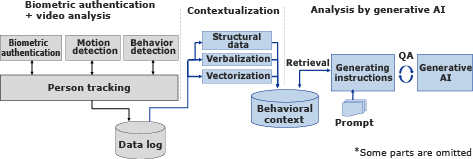
Such techniques enable LLMs to understand a series of behaviors and to infer the intentions of those behaviors as well as future behaviors. Based on the results, it is expected that it will be possible to provide support aligned with the intentions and to predict and prevent risks that go against the intentions.
5. Demonstration Experiment and Results
5.1 Recording of use cases and events
To verify the effectiveness of the aforementioned concepts, we conducted a demonstration experiment aiming to understand suspicious behavior in office buildings.
In this demonstration experiment, cameras were installed at eight locations in the office, including the entrance and elevator hall. NEC’s FieldAnalyst1) system for Scene Understanding, which is a high-precision recognition technology for diverse behaviors2), was used to detect behaviors and actions including entry, exit, and stay and to create behavioral event data. We also identified individuals by face recognition at the entrance (Fig. 3).
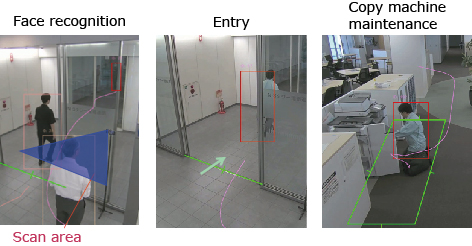
In this way, after detecting and recognizing the actions and behaviors of individuals, this data was converted into texts that stated who did what when and where for each event. By using general-purpose Sentence Similarity models, feature vectors corresponding to the meaning of texts were extracted and then recorded in a database.
5.2 Extraction and analysis of behavioral events
Next, at an appropriate time such as when an individual exited the office building, a series of events that occurred after that individual entered the building was extracted from the database. Then we converted those events to natural language texts and entered them into the LLM together with instructions, such as “Point out any behaviors in the following behavior records that seem unnecessary or suspicious in regards to the service provider’s tasks and tell me the reason,” to make inferences (Fig. 4).
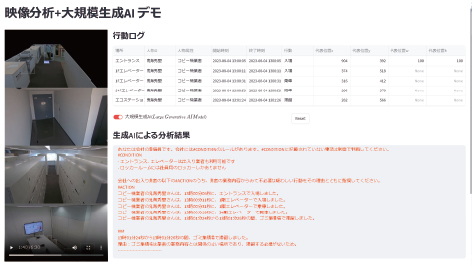
To understand any behavior from videos, it is usually necessary to define what behaviors fall under those that should be understood, create rules and learning data to describe the defined behaviors, and train the video analysis system to learn them. However, for behaviors that are difficult to strictly define such as those that are suspicious, it is impossible to create rules and learning data that cover all cases. By using the general knowledge that LLMs and LGAIMs have, we expect to help solve this challenge and achieve an understanding of behaviors at realistic costs. NEC verified the possibilities in this demonstration experiment.
5.3 Results of the inference by LLM
This section presents the characteristic results obtained from this demonstration experiment. In regard to a behavioral event where the cleaners entered the site where garbage is stored and collected the garbage, the LLM responded that there was nothing to point out. However, for a behavioral event where a copy machine service provider entered the site where waste was stored and searched for something, the LLM pointed it out as suspicious behavior, giving the reason that “the garbage storage site has nothing to do with the tasks of the service provider and there is no reason to stay there.” For another behavioral event where a non-management employee opened and closed a locker in the locker room, the LLM responded that, “there is nothing to point out.” However, in that same behavioral context if a cleaning service provider opened and closed a locker in the employees’ locker room, the LLM detected it as suspicious behavior.
In this way, we demonstrated that suspicious behaviors can be detected even in the privacy of an office building in the real world by combining the video analysis and LLM without defining rules or learning.
Meanwhile, when only using general knowledge, there may be cases where non-suspicious behaviors are detected or where suspicious behaviors are overlooked. For example, if cleaning equipment is stored in a locker, opening and closing the locker are necessary actions for the cleaners to perform their jobs but suspicious behaviors otherwise. NEC has confirmed that this challenge can be addressed by improving the ways the LLM is instructed, such as by including operation-specific rules in the prompts.
6. Conclusion
This paper presented the current situation and future potential of understanding behaviors in the real world by using video analysis and generative AI. NEC aims to use this technology to realize next-generation physical security that will contribute to the safety and security of people in the real world. In addition, this technology can be utilized to support operations in plants and warehouses, discover customer needs and dissatisfaction from customers’ online or offline behaviors, and for a variety of other purposes. So, NEC intends to promote the use of this technology in a variety of fields in addition to next-generation physical security.
While this technology has a great potential, many challenges remain. For example, the LLMs that are widely used mainly learn from information that is publicly available and collected from the Internet, books, and other sources. Therefore, they may not be able to understand behaviors exhibited in highly unique and confidential locations such as the special facilities of companies and government offices. To apply this technology to the real world, it is necessary to learn highly unique and confidential information of the real world while maintaining confidentiality. Also, if AI advances into understanding people’s behaviors, there are concerns that it may violate human rights or privacy invasion, so it is extremely important to operate in a way that complies with laws, ordinances, and social rules while applying technical protection.
By utilizing NEC’s cotomi3), a generative AI model developed by NEC that enables individual tuning by using proprietary data in a highly confidential on-premises environment or by taking similar measures, NEC will actively work to address these challenges and aim to achieve a society where people can show their humanity with a sense of security.
References
Authors’ Profiles
Senior Professional
Biometrics and Visual AI Platform Department
Professional
Biometrics and Visual AI Platform Department