Global Site
Displaying present location in the site.
Streamlining Doctors’ Work by Assisting with Medical Recording and Documentation
Vol.17 No.2 June 2024 Special Issue on Revolutionizing Business Practices with Generative AI — Advancing the Societal Adoption of AI with the Support of Generative AI TechnologiesIn this paper, we explain a technology that uses a large language model (LLM) to streamline doctors’ work by assisting with medical recording and medical documentation. For support in creating electronic medical records, speech of conversations between doctors and patients is recognized and then drafts of medical records are generated; for support in creating medical documents, a summary of the progress in treatment is created from medical records and then drafts of medical certificates are created for insurance claims, referral letters, etc. As a result of the improved efficiency, it is estimated in the model case that using LLM for support is expected to save 116 hours/doctor per year in the creation of medical records and 63 hours/doctor per year in the creation of medical documents. In the creation of medical documents, technological developments are further advanced, and a verification experiment has confirmed that the time spent on creating the documents can be halved. We will continue to develop technologies in preparation for the enforcement of work style reform for doctors in the revision of Japan’s Medical Care Act in April 2024 and aim to apply the technologies in other countries, such as India.
1. Introduction
The Work Style Reform Act was adopted in April 2019, but its application has been suspended for five years for some occupations including doctors and professional drivers. While the act is about to come into effect for doctors in April 2024, survey results show that approximately 20% of hospital doctors are at risk of death from overwork by working beyond the standard limit of 960 hours of overtime work per year1) and that urgent countermeasures are required.
Multifaceted preparations are being made to counteract the culture of overwork and include utilizing family doctors to avoid a concentration of work in large hospitals, shifting some of the doctors’ work to nurses and other healthcare professionals, and reducing workloads by utilizing information and communication technology (ICT) 2). As the speed of the evolution of ICT increases rapidly, large language models (LLMs) 3) 4) have attracted attention in recent years. However, there are no cases of actual application to medical services in Japan.
This paper presents a technology that uses an LLM to improve doctors’ work efficiency by supporting the creation of electronic medical records and medical documents.
Specifically, section 2 outlines the results of the analysis of the doctors’ work, and section 3 describes the technology that supports the creation of electronic medical records and medical documents. Section 4 explains an application to support the creation of medical documents—an area where the development of the technology has further progressed—and verification experiments utilizing this application. Section 5 describes a case in India to show the possibility of its application overseas.
2. Doctors’ Work
With the cooperation of Tohoku University Hospital’s Bedside Solution Program, Academic Science Unit (ASU) and the Department of Otolaryngology-Head and Neck Surgery, we observed doctors’ workplaces (outpatient clinics and hospital wards) from June to November 2022. Fig. 1 shows the proportion of time spent on each task performed by doctors, as obtained through on-site observation. Approximately half of the working time is spent on non-medical work such as the creation of electronic medical records and medical documents as well as schedule coordination. Furthermore, the results of the surveys in 10 other hospitals suggest that the creation of electronic medical records and medical documents does not require medical judgment, that people other than doctors can do these tasks, and that providing support for these tasks can be expected to produce a significant effect on reducing working hours.

The potential reduction in time was estimated to be 32 minutes/doctor per day for the creation of electronic medical records and 20 minutes/doctor per day for the creation of medical documents. If we assume that the actual time spent on these tasks was reduced 90% of the estimation, the annual overtime per doctor is expected to be reduced by 116 and 73 hours respectively. Also, labor costs are expected to be reduced by the doctors doing less overtime work. These analyses suggest that supporting the creation of electronic medical records and medical documents is expected to have a significant effect in reducing doctors’ working hours and improving hospital profits.
3. Support for Creation of Electronic Medical Records and Medical Documents
3.1 Support for creation of electronic medical records
On-site observations revealed that significant time savings are expected when interviewing outpatients, explaining medical conditions and treatment plans, and entering details of rounds and treatments in hospital wards into electronic medical records. Based on this, NEC’s technology recognizes speech of conversations between doctors and patients and then generates drafts that can be quickly revised by doctors during consultations. One of the challenges to realizing this functionality is that it is difficult to collect speech data of conversations in hospitals, making it difficult to train highly accurate speech recognition models.
Therefore, we adapted the conversational speech recognition model to the medical domain as shown in Fig. 2. We created synthesized speech of medical terminology from medical papers and trained the conversational speech recognition model to learn the vocabulary and to learn the tones of voice that cannot be covered by synthesized speech from actual speech of general terms. This will make it possible to recognize conversational speech at healthcare sites with a high accuracy.
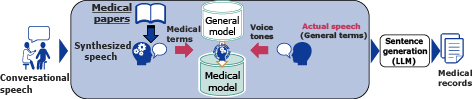
The aforementioned medical model and the general model that was trained using actual speech of general terms were compared by testing the recognition of speech data of explanations about medical conditions to outpatients. The speech recognition accuracy rate of the general model, which does not support medical terminology, was 75%, whereas the medical model achieved a significant improvement in speech recognition accuracy with a rate of 89%. By inputting these highly accurate recognition results into the LLM, it is possible to generate drafts that match the formats of the electronic medical records.
3.2 Support for creation of medical documents
On-site observations show that significant time savings can be expected in the creation of medical documents that require a summary of a treatment’s progress such as referral letters to other hospitals, medical certificates to be submitted to insurance companies, and discharge summaries of hospitalized patients. Therefore, NEC’s technology summarizes the treatment’s progress from electronic medical records and generates drafts that can be quickly revised by the doctors. One of the challenges to realizing this functionality is to understand and summarize the context of on-site medical records containing many technical terms and their abbreviations.
To meet this challenge, we generated summary texts based on context understanding in the steps shown in Fig. 3. In the first step, the meaning of words (e.g., test and medication) is estimated from the medical record’s context, and the progress of the treatment is extracted. This was achieved by utilizing a unique database that was built using medical records accumulated over a 10-year period at Tohoku University Hospital and by using a trained medical language model. In the next step, the LLM fills in omissions, shapes the texts, and generates sentences that follow the format of medical documents. These steps enable the generation of high-quality medical documents. Even if the generated sentences contain incorrect information, they can be easily checked and corrected.
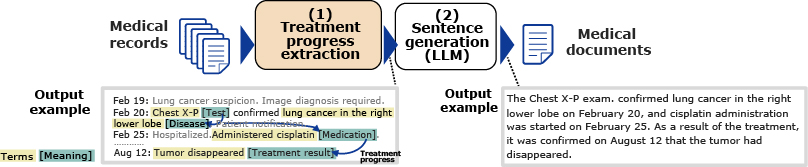
We evaluated the accuracy for estimating the meaning of medical terms by using data from on-site medical records written by doctors5). The terms whose meaning was to be estimated included time-related expressions, medical conditions and symptoms, treatments, tests, medications, clinical contexts, locations, changes, and characteristics. We achieved a high average rate of estimation accuracy at 90%. Section 4 describes the prototype application to provide support for the creation of medical documents and its evaluation.
4. Application to Support Medical Document Creation
We created a prototype application to support the creation of medical documents and verified the results of using the application to reduce time spent on creating documents.
4.1 User interface
Fig. 4 shows a screenshot of the prototype application’s user interface (UI) that supports medical document creation. The UI consists of three columns: the left, the center, and the right. The left contains the information entered from the electronic medical records. The center displays the results of the application’s estimation of the medical terms from those records in a table format. The rows of the table represent time, and the columns represent meanings. The upper part of the right column outlines the treatment progress that was extracted from the estimated meanings as bullet points. In addition, the lower part of the right column displays a text summary generated by the LLM4) based on the extracted treatment progress.
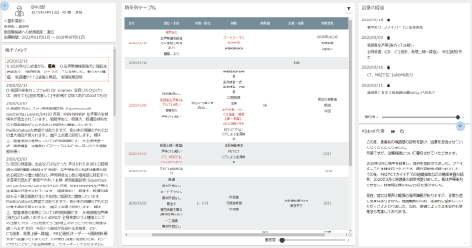
Compared to reading voluminous electronic medical records extending over several years in the longest cases, doctors can readily understand the treatment progress by viewing the table in the center column. Furthermore, the LLM generates texts summarizing the treatment progress. By doctors then checking these texts and confirming that they are correct, documents can be readily created.
4.2 Verification of effectiveness
From October to November 2023, an experiment to verify the effectiveness of the prototype application was conducted with the cooperation of 10 doctors from the Department of Otolaryngology-Head and Neck Surgery at Tohoku University Hospital. We measured how much time is saved when creating a referral letter with the help of the prototype application compared with creating a referral letter only from the contents of electronic medical records without the support of the prototype application. As a result, it was confirmed that the time required to create a referral letter was reduced by 47% with the use of the application (reduced from 16 minutes 15 seconds to 8 minutes 40 seconds on average). As a result, a doctor who spends a similar proportion of time on tasks as in Section 2 is expected to spend 63 fewer hours annually on creating medical documents.
5. Case in India
India suffers from a chronic shortage of healthcare professionals. The number of doctors per 10,000 people in India is only eight compared to 20 in Japan, and many have high expectations for the use of IT to enable fewer doctors to treat more patients. Large hospitals have already introduced the Healthcare Information Management System (HIMS); however, it is difficult to say that it has been sufficiently effective. Through on-site surveys and interviews with doctors and management teams at several hospitals, NEC understands the challenges and proposes solutions.
5.1 Challenges
The following challenges were observed in hospitals that had already introduced the HIMS:
- Doctors have no incentives to use the HIMS.
- The complicated UI has resulted in minimal use, if at all.
This is presumably because the final decision on using the system in India is left to the on-site doctors rather than managers. As a result, hospitals that have already introduced the HIMS have all the information entered in the Note fields as text in random formats, such as text containing abbreviations, rather than a standardized format and they use printed paper medical records when referring to past records. Therefore, their involvement in improving efficiency is limited.
5.2 Solution
NEC drafted a solution to automatically extract necessary information for the HIMS without changing the format of the doctors’ daily consultations. In this solution, doctors are requested to enter text in the Note field as usual, but past consultation summaries and chronological test information are automatically systemized by using the natural language processing technology. This would reduce the time required by doctors to extract information from paper medical records and allow them to reduce the individual patient’s consultation time.
Fig. 5 shows a screenshot of the HIMS prototype in progress. On the left, doctors enter text in whatever format they wish to use during consultations; on the right, information resulting from the use of the natural language processing technology is displayed. The medical condition of the patient, diagnostic results, and prescription details recorded by the doctor during the consultation are automatically entered in the respective fields in the HIMS through the use of the natural language processing technology and are displayed in the relevant fields on the right side of the screen. In addition, a table of chronological events and a summary of past diagnoses summary are automatically generated by the LLM4) and displayed in accordance with the past consultation data entered in the HIMS.
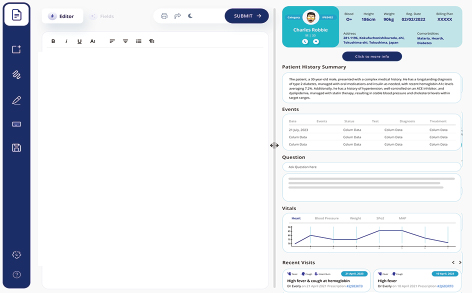
6. Conclusion
This paper introduced technology that streamlines doctors’ tasks by supporting the creation of electronic medical records and medical documents. Observations at medical sites revealed that support for the creation of electronic medical records and medical documents may have a significant effect on reducing working hours and improving hospital profits. We also described the challenges in realizing these support technologies and the means to solve them. Regarding the support for the creation of medical documents—where the development of the technology has been leading the way, this paper presented the results of a demonstration experiment that confirmed the time used to create the documents could be halved. We will proceed with the development of this technology in preparation for the enforcement of the work style reform for doctors in the revision of Japan’s Medical Care Act in April 2024. In addition, this paper also presented challenges unique to India as an overseas case study and solutions to them. We will continue to proceed with service development in cooperation with relevant departments in Japan.
References
- 1)
- 2)
- 3)
- 4)
- 5)
Authors’ Profiles
Manager
Biometrics Research Laboratories
Deputy General Manager
NEC Laboratories India
Senior Data Scientist
NEC Laboratories India