Global Site
Displaying present location in the site.
Development of Neoantigen — Targeted Cancer Vaccine Therapy
Cancer immunotherapy is expected to be the fourth cancer therapy after surgery, chemotherapy and radiation treatment. Under this trend, NEC Corporation realizes that the mutation of cancer varies depending on individual patients and has thus proceeded to develop vaccine therapies targeting the tumor-specific antigens (hereinafter “neoantigens”) of each patient as cases of individualized medicine. This paper introduces the technique for identifying the neoantigen that can induce the strongest immune response of each patient based on effective use of genome analysis and AI technologies. Clinical trials of an individualized cancer immunotherapy using the technique introduced here have already been started.
1. Introduction
Cancer therapies can be broadly classified into the following three methods: “surgical therapy” that removes cancerous lesions; “chemotherapy” that destroys or reduces the growth of cancer cells using anticancer drugs and “radiation therapy” that irradiates cancer lesions to destroy the cancer cells. Each of them has advanced through a long history but still presents advantages and disadvantages even at present. In addition to the three therapies, “cancer immunotherapy” has recently been attracting attention as the fourth therapy (Fig. 1). This therapy originally controls the growth and progress of cancer by enhancing the body’s innate immunity to cancer. It is expected to be a treatment that can act throughout the whole body, provide sustained effects and present low side effects. In the rest of this paper, chapter 2 outlines the mechanism of cancer immunity and cancer immunotherapy, chapter 3 describes NEC’s approach toward cancer vaccine therapy, and chapter 4 introduces the neoantigen prediction method used in the development of vaccine targeting tumor-specific antigens (hereinafter “neoantigen”).
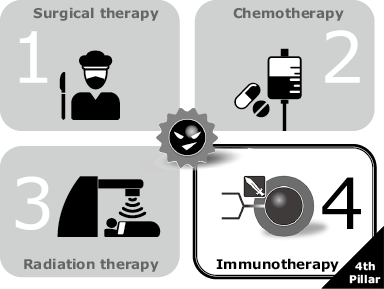
2. Mechanism of Cancer Immunity, Cancer Immunotherapy
Genes are damaged by various contents of tobacco and food such as carcinogenic substances and active oxygen, so mutated cells are produced every day in the body. The immune system protects the body by surveilling and eliminating abnormal cells, but cancer could be formed with the help of the cancer escape mechanism if cancer cells slip through the immune attack and proliferate and the body can no longer maintain immune dominance. Fig. 2 shows the induction of immune response to the cancer cell. Cancer cells release cancer antigens on their cell surfaces (Fig. 2-1). The released cancer antigens are phagocytized by antigen presenting cells such as dendritic cells, which then migrate to lymph nodes through the lymph ducts and place the peptides, which are fragments of the cancer antigens, on the major histocompatibility complex (MHC) class I and II molecules to present them to T-cells (Fig. 2-2). The T-cell recognizes the cancer-antigen peptide presented on the MHC molecules by means of the T-cell receptor (TCR) and activates itself (Fig. 2-3). The activated T-cells migrate through the blood vessel toward the cancerous tissue (Fig. 2-4) and then exit from the blood vessels to infiltrate the cancer cell tissues. The cancer cells also present their own cancer-antigen peptide on the MHC molecules so the T-cells recognize the cancer-antigen peptide/MHC complex via TCRs and attack/eliminate the cancer cells (Fig. 2-5).
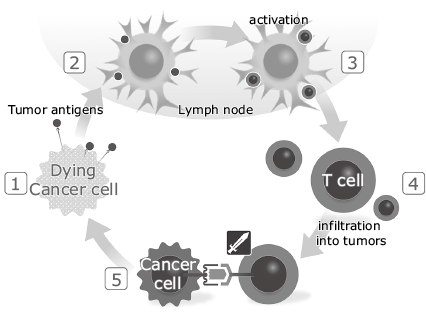
Cancer immunotherapy has variations including the following: “BRM therapy (immunomodulating therapy),” the oldest immune therapy method administering a foreign object into the body to activate the immune response; “immune cell therapy,” in which immune cells such as lymphocytes and dendritic cells are removed from the patient’s body, cultured in vitro and returned into the body; “cancer vaccine therapy,” that injects part of the cancer antigen; “gene-modified T-cell therapy,” that extracts the T-cell from the patient’s body, modifies the gene so that the cancer cell can be recognized, cultures the T-cell and returns it into the patient’s body again; “immune checkpoint therapy,” which inhibits transmission of immunosuppression signals by coupling with the molecule used by the cancer cell to brake the functioning of immunity and inhibit the attack of immune cells.
3. NEC’s approach to cancer vaccine therapy
Among cancer immunotherapies, NEC is focusing on cancer vaccine therapy. Recent advancement of next-generation sequencing and bioinformatics has made it clear that mutation is extremely variable among patients. NEC has therefore started development of cancer vaccine therapy as an instance of individualized medicine, which targets the neoantigen of each individual patient1). The vaccine development process conceived by NEC involves harvesting tumor and normal tissues from the patient, and obtaining the whole exome sequencing data and RNA sequencing data using next-generation sequencing. The obtained sequencing data and RNA sequencing data are analyzed by using an originally-developed neoantigen prediction system to select the neoantigen, and then synthesized with vaccine that incorporates the neoantigen. Finally the synthesized vaccine is administered to the patient (Fig. 3).
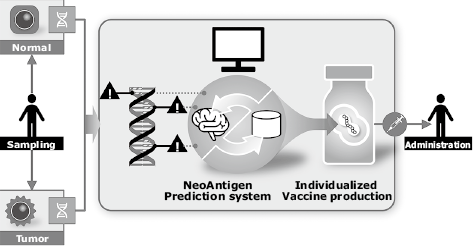
4. NeoAntigen Prediction Technique Developed by NEC
Fig. 4 shows the processing flow of the neoantigen prediction technique developed by NEC. First, the whole exome sequence (WXS) data of tumor and normal tissues are mapped onto a reference genome sequence. Tumor-specific mutations are detected by comparing the results of each. Peptides with nine residues containing this mutation are used as candidate epitopes. Also, the WXS data of the normal cell is used to identify the HLA type.
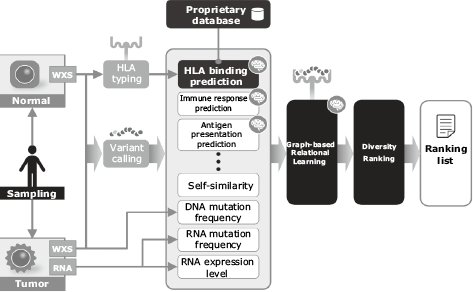
Next, the scores associated with the immune responses to the candidate epitopes are calculated. These scores include the following prediction values: machine learning predictions of the binding affinity between the candidate epitope and HLA molecule2), machine learning predictions of whether or not the candidate epitope will elicit an immune response, machine learning prediction of whether or not the candidate epitope will be presented on the cell surface. These scores also include self-similarity, mutation frequency, the RNA expression level, and so on. Then the total score is calculated by means of a graph-based relational learning3) out of multiple scores mentioned above for each candidate epitope. In graph-based relational learning, a graph consists of vertices representing epitopes and edges which connect two vertices with high similarity (Fig. 5). Next, the score vectors of the candidate epitopes assigned to the vertexes are converted into dimensionally compressed vectors (embedded vectors) using a deep neural network. The parameters of the deep neural network are retrained based on the difference between the embedded vectors calculated on each vertex and those calculated on the adjacent vertices. This processing is repeated until the differences converge, and the embedded vector is obtained (Fig. 6). The embedded vectors are used as the feature to obtain the total immune response prediction score, and then the size of each embedded vector is changed to the size of the predicted score (Fig. 7). The diverse epitopes with large scores are then selected from the embedding space in order to select the vaccine candidates.
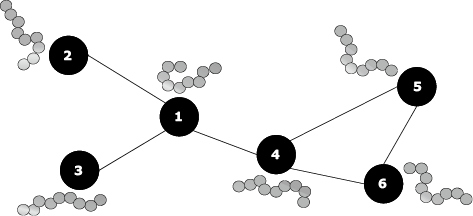
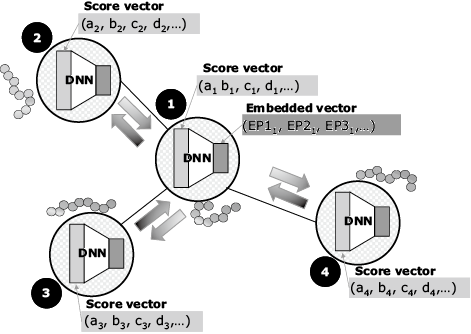
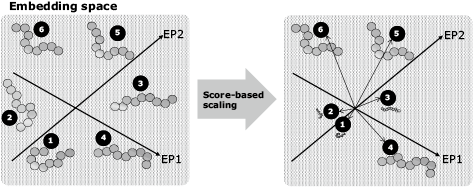
5. Conclusion
In this paper, the authors described three main subjects: the mechanism of cancer immunity and cancer immunotherapies, NEC’s approach toward cancer vaccine therapy, and the neoantigen prediction technique developed by NEC. This technique is not only applicable to individualized cancer vaccines but also to the design of anti-COVID-19 vaccines4).
The clinical trials of an individualized cancer immunotherapy using this technique have already been started. In the future, the data accumulated through the trial period will be verified to see the types of patients for whom the therapy is effective. For patients who find the therapy effective, we shall look for biomarkers that can be useful in treatment planning, such as how long it should be administered. NEC is determined to tackle the drug development business and hopes for success of the present clinical trial as well as the approval of the cancer vaccine as a pharmaceutical product to be delivered to many patients.
Reference
- 1)
- 2)Kuksa Pavel P. et al.: High-order neural networks and kernel methods for peptide-MHC binding prediction, Bioinformatics, Volume 31, Issue 22, pp.3,600-3,607, July 2015
- 3)Alberto Garcia-Duran and Mathias Niepert: Learning Graph Representations with Embedding Propagation, NIPS 2017 Proceedings of the 31st International Conference on Neural Information Processing Systems, pp.5,125-5,136, December 2017
- 4)
Authors’ Profiles
Senior Manager
AI Drug Development Division
Ph. D.
Assistant Manager
AI Drug Development Division
AI Drug Development Division
Senior Researcher
Systems and Machine Learning, NEC Laboratories Europe
Ph. D.