Global Site
Displaying present location in the site.
How Face Recognition Technology and Person Re-identification Technology Can Help Make Our World Safer and More Secure
Core Technologies and Advanced Technologies to Support BiometricsTo boost security and enhance convenience, many public facilities such as airports and train stations are beginning to roll out new face recognition technology, with many more already planning to implement it. On a more mundane level, this new technology is poised to replace passcodes and ID cards in applications such as payment verification, mobile phone access, online shopping, and so on. In this paper, we will look at the face/human body detection and face matching technologies introduced by NEC, as well as person re-identification technology which complements face recognition. Person re-identification technology is another form of biometrics that can identify an individual based on their overall appearance, even when their face is not visible. We also review the test results of the Face in Video Recognition (FIVE) program conducted by the United States National Institute of Standards and Technology (NIST) in which NEC was ranked first in evaluation accuracy.
1. Introduction
Over the past couple of decades, the world has suffered numerous indiscriminate terrorist attacks represented most spectacularly by the September 11 attacks and Boston Marathon bombings. Growing public anxiety has led to ever-increasing demands for stronger and more reliable security measures, including the implementation of face recognition technology. NEC’s face recognition technology ranked first in benchmark testing held by the United States National Institute of Standards and Technology (NIST) in 2009, and has topped the rankings three times since then1). NEC also was ranked first in the NIST’s Face in Video Recognition (FIVE)2) in 2017 (Fig. 1).
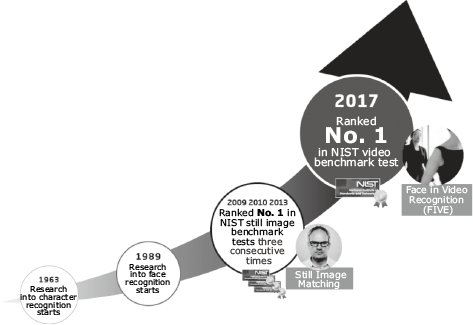
NEC’s face recognition products have been adopted in more than 100 systems in 45 countries the world over. They have been utilized at major facilities around the world, including New York’s John F. Kennedy Airport, the main airports in Brazil, various Australian government organizations, and the South Wales Police. In 2014, the NEC Group, in collaboration with TAPIRS Co., developed the first ticket ID system in Japan to use face recognition software for concert ticket sales. Applied to 1.1 million people over a period of four years, this system has made it much more difficult to gain admission with illegally resold tickets3).
As effective and widely deployed as face recognition is, it often needs to be supported by other biometric recognition technology to ensure reliable identification in situations where the face cannot be properly seen. We all know that we can usually identify a friend or family member even when they are facing away from us thanks to their overall appearance. Similarly, person re-identification technology can identify an individual based upon the whole-body image of that individual. In combination with face recognition technology, person re-identification technology tremendously enhances identification capabilities under almost any circumstances.
In this paper, we introduce our face recognition and person re-identification technology in Chapter 2, summarize the evaluation results of the FIVE conducted by the NIST in Chapter 3, describe some usage scenarios for person re-identification in Chapter 4, and offer our conclusion in Chapter 5.
2. Face Recognition Technology and Person Re-identification Technology
Rapid advances in deep learning technology over the past few years have helped to increase the power of face recognition and person re-identification technology, leading to dramatic improvements in the precision of identification capability. In this section, we will introduce the main features of face/human body detection technology, face matching technology, and person re-identification technology — the core technologies driving this quantum leap in identification precision.
2.1 Face/human body detection technology
Face/human body detection technology is used to spot faces and human bodies in images. The deep learning technology constructs convolutional neural networks that output the positions of the faces and human bodies captured in input images. Due to differences in individual posture and clothing, human bodies can present near-infinite variations in appearance, making them much more complex than faces. To deal with this complexity, a massive number of images of the human body — showing an enormous variety of individuals engaged in activities such as walking and running — is input into the system as learning data. This makes it possible to ensure detection of faces and human bodies in various settings (Fig. 2).
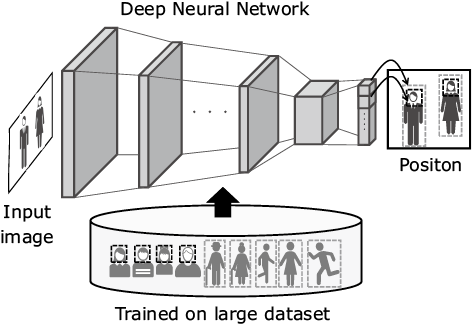
2.2 Face matching technology
Face matching technology compares various normalized feature points — including position, size, and angle — in a person’s face with the face in the registered image. The normalized face image is input into a convolutional neural network based on deep learning to extract the optimal features necessary to accurately identify an individual. This makes the system less susceptible to recognition problems caused by partial occlusion, aging, wearing a mask, etc. The result is more robust individual identification performance despite changes in appearance (Fig. 3).
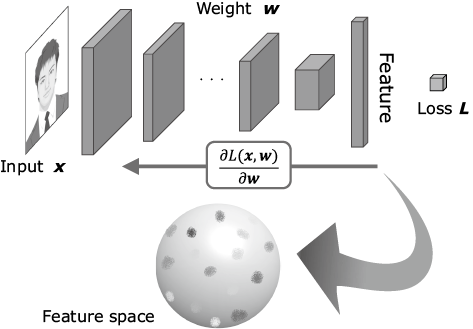
2.3 Person re-identification technology
As with face matching technology, person re-identification technology is also used to determine whether an individual is the person in gallery or not, so the basic function of these two technologies is the same. The difference is that person re-identification technology uses whole-body images as the basis for identification, rather than just face images. In this case, an image of an individual’s entire body is input into a deep-learning network, and the feature vectors are output. The feature vectors in the gallery image are then compared with those of the individual and a similarity score is calculated. On this score, whether the subject is the person in gallery or not is determined (Fig. 4).
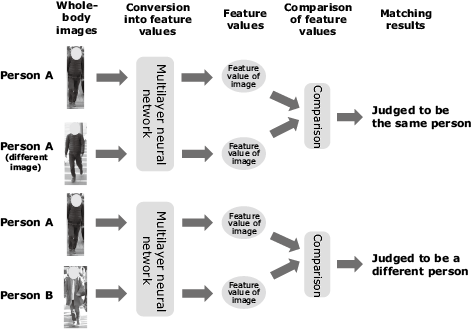
One of advantages of our person re-identification technology is that it can still be effective even when the body is partially occluded (Fig. 5). To achieve this capability, NEC incorporated special procedures in the learning method used by the deep learning system.
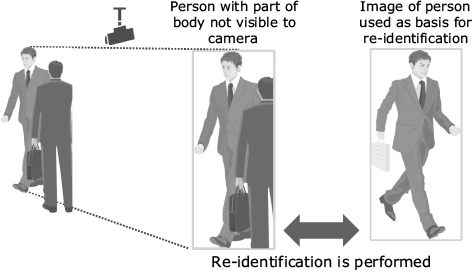
3. Evaluation of Our Video Face Recognition Performance by the NIST
In this section, we discuss the results of tests conducted on NEC’s video face recognition technology by the NIST, which were made public in 20174). Called Face in Video Recognition (FIVE), this test is designed to evaluate the performance of face recognition technology in video images and is a precision test intended for subjects who are not aware that cameras are tracking them. The tests started in February 2015, and the final report was published in March 2017. There were 16 participants from around the world. For the purposes of the test, it was assumed that the face recognition technology would be used for building access control and detection of suspected individuals. Tests were conducted under various scenarios (number of individuals registered, camera locations, number of cameras, etc.).
The outline of the test performed on the assumption of walkthrough recognition is shown in Fig. 6. This test was performed assuming that the setup was for walkthrough recognition at an airport or similar facility and that each individual would be recognized while unaware of the camera and without stopping in front of the camera. 480 people were registered in advance — equivalent to the average number of passengers per aircraft. The subjects to be recognized were in 248 video clips, and the evaluation was based on the highest matching rates. In this test, the faces were close to the camera, so it was a favorable environment for video face recognition.
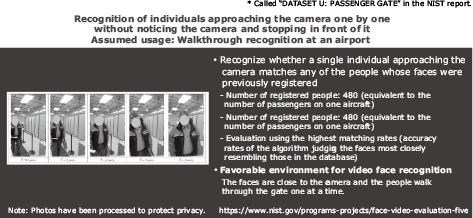
The results of the test are shown in Fig. 7. At 0.8%, NEC boasted the lowest error rate by far, less than a quarter of the rate of the second-ranked participant. The results made it abundantly clear that NEC’s system was capable of delivering reliable performance in a real-world environment where people are not aware of the camera and do not stop in front of it.
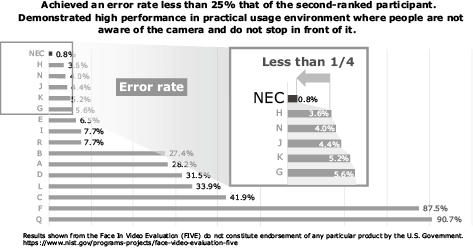
Now let’s look at the test to recognize people from images shot with outdoor cameras (Fig. 8). In this test, the subjects were far from the cameras and the subjects faced in many different directions, resulting in an adverse environment. Again, 480 people took part in the test and the video recording used for matching was about 133 hours long. Since the distances between cameras and individuals were significant, the faces were captured in low resolution. Moreover, the directions of the faces were varied. All these factors contributed to creating an unusually adverse environment for video face recognition.
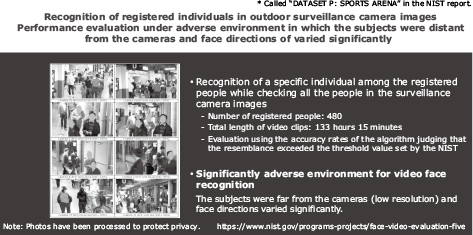
Fig. 9 shows the test results. NEC’s system proved able to detect more than 85% of the people with face recognition only. Our error rate was less than half of that of the second-ranked participant, demonstrating that NEC was the only vendor able to achieve reliable matching with low-resolution images.
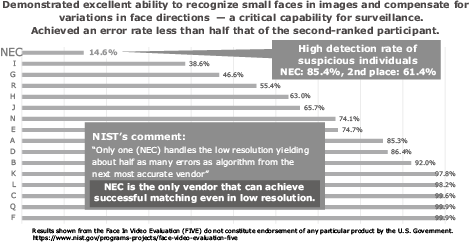
As we have seen, NEC’s face recognition technology has not only been highly assessed with still images, but also with video images. These tests clearly demonstrated that our technology could achieve stable performance under adverse conditions using either still or video images. This remarkable achievement has helped solidify our reputation as one of the world’s leading innovators in the field and inspired adoption of our face recognition technology in various projects around the world.
4. Usage Scenarios for Person Re-identification Technology
One possible usage scenario for person re-identification would be to locate a missing child or other specific individual in a large facility such as an airport. Most large facilities have numerous cameras installed at various locations. Using the images captured by these cameras, the user can search for people. While face recognition may be effective for searches of specific individuals in this situation, it cannot be used to identify people whose faces are not visible because they are facing away from the camera, for example. In this case, searching the resulting video footage with face recognition alone will be ineffective, delaying locating the missing person.
One solution to this problem is to search for a person using person re-identification software while coordinating multiple cameras. Thus, when a person’s face is visible in the image from one camera, that person can be identified using face recognition technology. Subsequently, the person re-identification technology can be called into play to interrelate images from multiple cameras (Fig. 10), enabling the search to be continued even when faces cannot be seen in images. By comparing the ongoing search results of people with the linked face recognition data, the person re-identification software makes it possible to execute a search for an individual at any given moment.
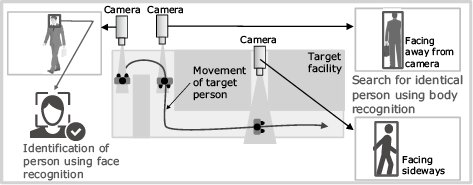
In addition, even when not combined with face recognition, search using person re-identification can detect traffic lines of people in images, making it possible to detect individuals who are loitering or otherwise acting suspiciously.
5. Conclusion
In this paper, we have discussed NEC’s face recognition and person re-identification technologies and summarized the NIST test results. The potential market for systems that combine face recognition and person re-identification is increasing year by year. In order to put this combination in practical use, it is important to take requirements for both security and privacy into consideration. We at NEC are committed to promoting technological development that ensures individual privacy in keeping with the relevant laws and regulations of respective countries, while enhancing our product’s performance in terms of security by further improving recognition precision.
Reference
- 1)
- 2)
- 3)
- 4)
Authors' Profiles
Principal Researcher
Biometrics Research Laboratories
Biometrics Research Laboratories
Assistant Manager
Biometrics Research Laboratories
Assistant Manager
Biometrics Research Laboratories
Research Fellow & Director
Biometrics Research Laboratories