Global Site
Displaying present location in the site.
PoC of AI Demand Forecast Deployment in the NEC Group’s Manufacturing Facilities from an Ethnographical Perspective
This paper describes our proof-of-concept (“PoC”) activities of demand forecasting. NEC is challenging the prediction of server demand using AI technology in order to prevent parts stock-outs at the same time as reducing the stock inventory at the Kofu Plant of NEC Platforms, Ltd. In order to render the demand prediction convincing for use, heterogeneous mixture learning, which is an NEC-original AI technology, is being applied so that the grounds for prediction can be presented together with the results of prediction. In addition, ethnography is adopted in the study technique with the aim of identifying the most effective situation for which the results or grounds for prediction can encourage decision making and for designing display styles.
1.Introduction
In the IoT era, in which everything is connected to the Internet, the effective use of the large amount of collected data is greatly anticipated. Some production sites have already made various efforts in data utilization. For example, since October 2015 the NEC Group implemented IoT at the Fukushima Plant, NEC Platforms, Ltd. (previously the main plant of NEC Network Products, Ltd.) and has thereby achieved a 20% productivity improvement.
NEC Business Creation Division has been promoting active utilization of data within the NEC Group as a critical challenge in the IoT era. As one of its activities, the Kofu Plant of NEC Platforms, Ltd. (“the Kofu Plant”) is undertaking a PoC investigating product demand prediction using AI for parts inventory optimization. In this paper, the authors will report on the issues deriving from this PoC by focusing on the possibility of deployment and utilization of prediction at the production sites and the relevant measures.
2.Outline of Business Operations at the Kofu Plant, Issues Related to the Parts Inventory
The Kofu Plant is producing a large variety of products including supercomputers, PC servers, ATMs and power storage systems. Among these, most of the PC servers are made to individual orders according to customer specifications. The system coordinates order receipts, production and shipments and enables each customer to choose configurations from more than 100,000 options and receive the product within “minimum 4 operating days” from the order receipt.
For parts and materials procurement, the plant adopts a step-by-step ordering system for which the requirements plan (forecast) is presented to the supplier in advance and the actual order is placed immediately before the production starts. The requirements forecast is reviewed each week from six months before until immediately before the final order (rolling planning) and the review results are presented to the supplier. In addition, the “shipment within minimum four operating days” presupposes that the required parts and materials are available at the time of production. Consequently, since there are lead times for parts and materials even at the final order stage, it is required to place the order in anticipation without the actual order receipt. As seen here, currently, it is always necessary to estimate the forecast either when planning or ordering parts. For estimating the forecast of parts at high accuracy, the production plan must be undertaken accurately.
The production management department that makes these decisions has been procuring generous amounts of parts each time to prevent sales opportunity loss due to parts stock-outs. However, the company management has seen this practice as requiring modification because the inventory balance is deteriorated due to the excessive inventory. The production management department needed to tackle this issue to balance “prevention of parts stock-outs” and “reduction of parts inventory”. The present PoC using AI for the product demand prediction was conducted to solve this issue.
3.AI Techs Used in PoC
The PoC used “heterogeneous mixture learning,” which is one of NEC’s AI technologies called “NEC the WISE.” The heterogeneous mixture learning technology automatically finds regularities with high accuracies from various data and performs optimum predictions according to the current circumstances based on found regularities. This procedure generates accurate prediction even in the complicated cases requiring too many trial and error attempts if done by humans. Another feature of this technology can indicate the grounds for prediction in an easy-to-understand manner that enables confirmation of the reasoning behind it.1) A previous study found that, it is important that the effectiveness can be judged in the context of convincing results in applying the results of data analysis to human decision making.2) As the aim of the present PoC is to support “human decision making” in production planning for parts inventory optimization, we have adopted the heterogeneous mixture learning as the analysis technology due to its capability of presenting the grounds for prediction.
Using this AI technology, we then set the in-house data, such as sales data, and the external data including calendar information and macro economy indices as the learning environment and predict the demand for each product for each month from 3 to 6 months ahead.
4.Issues of Demand Prediction
Although we have been providing demand predictions and their reasons to the Kofu Plant since 2015, they were not used in the actual production plan. The main reason was that “prediction” had never been used in management at the Kofu Plant. As a result, an effective situation of “demand prediction” usage could not be identified.
It is said that human action is determined by circumstances.3) Naturally, utilization of demand prediction should be made according to the current situation. In order to promote active use of new types of information such as “demand prediction” for production planning, whose protocols are usually more or less fixed, we assumed that identification of a suitable situation of information usage in the production planning was crucial as the first step. Furthermore, it has also been pointed out that the use of demand prediction in the manufacturing industry is influenced by issues in the organizational structure or in operations and identification of the effective situation is seen as also being essential.
Moreover, it is also necessary to design an optimum display style beyond a simple indication of collections of predicted figures for use in predictions, which may sometimes be contrary to human intentions in decision making.
We have therefore decided to define the most effective situation of “demand prediction” in the “decision making” and to then examine display style.
The technique “ethnography” we adopted in this PoC has traditionally been used in the cultural anthropology discipline. With this technique, the anthropologist “experiences” a subject culture to understand it from the insider’s view point. The method gradually came to be applied to business activities as a means of obtaining deeper understanding of the customers4) and recently new business opportunities have been found in observations based on many case studies. Following this trend, we have decided to adopt “ethnography” to understand the production plan operations before proceeding to challenge identification of a suitable situation and the design of the display style.
5.Ethnography in Demand Prediction
Interviews conducted in advance have clarified the overall operation flow as shown in Fig. 1.
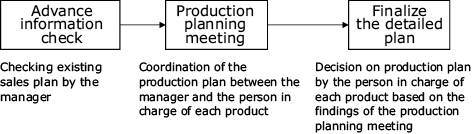
Based on the flow, we have decided to conduct the ethnographic study by dividing it into two steps.
Step 1: Identification of the situation for applying prediction results
Assessment of the performance of the person in charge and activity observations are conducted for each operation to understand what kind of operations are executed and the reasons behind the choice. In this process, the most effective situation of the “prediction results” in the “decision making” of the production plan is identified.
Step 2: Determination of an optimum display style
The optimum display style for the situation of decision making is planned. It is subjected to improvement by observing how it is to be actually put into practice.
6.Results, Considerations
After having an interview with the manager in advance on the overall flow, we performed operation observations of the “production planning meeting” and “finalize the detailed plan” by the two persons in charge of actual operations, for a total 120 minutes. We were then able to sort out the factors affecting the production plan based on the results of the interviews and observations. Fig. 2 shows the connections between the factors in the overall flow.
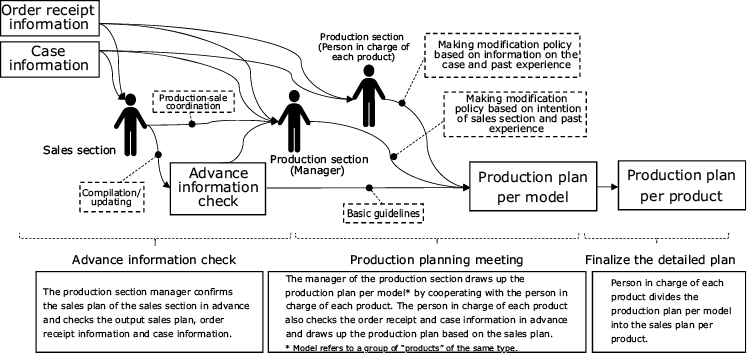
The process from the “advance information check” until the “production planning meeting” was influenced by various data and considerations, these were collected in the “production plan coordination meeting,” and the results were handed over to “finalize the detailed plan.” As it was found that “finalize the detailed plan” is defined to be the place of final decision making, in fact it is also a place of coordination based on the output from the “production planning meeting.” We therefore concluded that it was not the place where the plan could be modified significantly by demand prediction. Instead, it was also found that the “production planning meeting” is a place where the attendees make decisions based on shared information. We concluded that the “production planning meeting” where thoughts are mostly reflected, is the most effective situation for presenting the demand predictions.
We also decided to design the optimum “prediction display style” based on meetings. We observed actual applications of the designed prediction display styles three times at the production planning meetings, each of which lasted for 60 minutes. Fig. 3 and Fig. 4 show the results of improvements applied to the styles according to the findings of the observations.
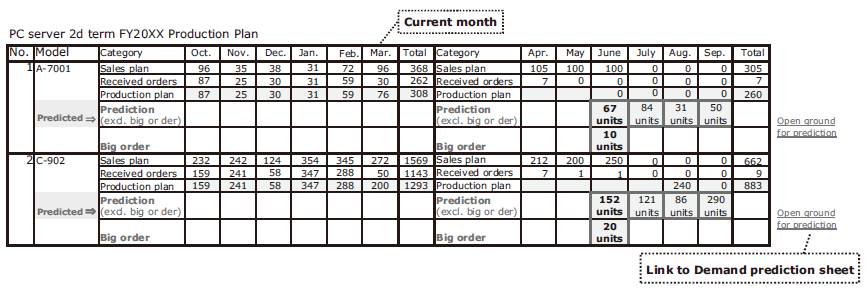
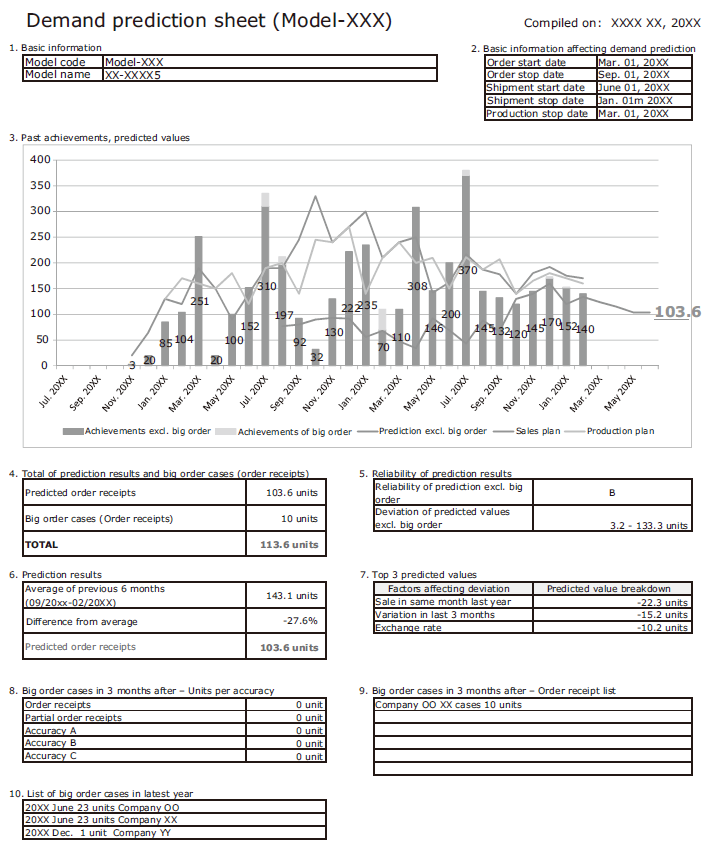
Fig. 3 is the template for the production plan that was the output from the meeting. It shows the predicted values directly so that the viewer can refer to the plans drawn up by others and from past achievements simultaneously with the prediction by machine learning. The link to the material named “Demand prediction sheet” indicating the grounds of prediction is embedded on the right side of the table for quick reference. The “Demand prediction sheet”, Fig. 4, shows past achievements and any deviation in the current predicted value of past trends, as well as the factors causing the deviation at a glance.
At the actual meeting, as shown in Fig. 5, it was confirmed that each predicted value could be accepted as it was if it was within the range of estimations by the attendants (Pattern A). When it was out of the range of estimation, it was accepted if it was convincing as “the value that should be” (Pattern B). If it was “a value not convincing for the attendants” (Pattern C), the final decision was made by opening the demand prediction sheet to confirm its grounds. In any case, it was confirmed that the demand prediction could exert influence on the production plan as one of the items of reference information.
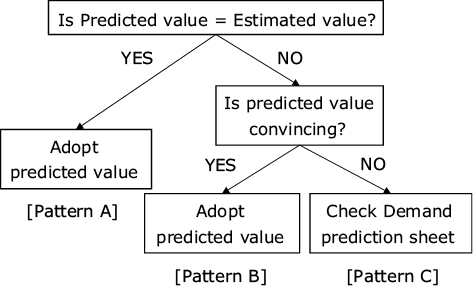
7.Conclusion, Future Perspectives
As clarified in the PoC, active prediction usage in the operations at the production sites, it is indispensable to optimize the “presentation of predictions” for each customer’s operations as well as to improve the accuracy of the predictions. For this purpose, we have attempted to increase the acceptance of demand prediction in the site operation, through specifying suitable situations for prediction and providing the results together with grounds for the prediction. Observation study as an ethnographical approach was taken for specification of suitable situations and display style design and “heterogeneous mixture learning” was employed for clarification of grounds for prediction as AI technology. We have employed respectively the “ethnography” focusing on observation study for the prediction situation identification and grounds display style design, and the “heterogeneous mixture learning” AI technology for the clarification of the grounds for prediction. The demand prediction can thereby be information “that can exert influence on the production plan.” At NEC, we are preparing for utilizing demand predictions as support for decision making in production planning. We believe that this strategy will realize the procurement that can obtain just sufficient quantities of materials and parts and fulfil the needs of achieving both “parts stock-outs prevention” and “parts inventory reduction” at the production sites.
The IoT era in which everything is connected to the Internet is expected to make more information available in a timely and efficient manner, which is currently unavailable to human labor. We are planning to utilize accurate prediction with AI technology and information from the IoT environment. In future, we will strive to offer a total mechanism to support decision making by providing relevant information for the production and design of the process.
Reference
2) K. Umezu, Y. Motohashi: A Study on Utilization of Analysis Results in Business and Interpretation of Model, Proceedings of the 30th Annual Conference of the Japanese Society for Artificial Intelligence, 3K3-4 (in Japanese), 2016
3) L. A. Suchman: Plans and Situated Actions, Cambridge University Press, 1987
4) M. Nakatomi, S. Iga, A. Shimada: Field research methods for understanding customers’ needs: Proposal of human-centered R&D process, Social Informatics Fair 2005 Workshop on Analytical Methodologies for CMC and HCI Studies, pp.4-8 (in Japanese), 2005.9
Authors’ Profiles
WASHIDA Azusa
Assistant Manager
Business Creation Division
NAKATOMI Masashi
Manager
Business Creation Division
YOSHITAKE Nobuhiro
Senior Manager
Business Creation Division
UMEZU Keisuke
Principal Researcher
Data Science Research Laboratories
YAMADA So
Data Science Research Laboratories
MOTOHASHI Yousuke
Senior Manager
AI Analytics Development Division
OSADA Tsukasa
Manager
Production Control Department
Kofu Production Management Division
NEC Platforms, Ltd.