Global Site
Displaying present location in the site.
Warehouse Staffing Optimization Solution Using Autonomous and Adaptive Control — NEC’s latest AI technology
In recent years, the pace of operations in the distribution industry has accelerated as demand for more frequent deliveries of smaller lots has become the norm. This has increased the complexity and difficulty of delivery tasks, leading to more demanding workloads and intense time pressure. With the availability of labor simultaneously decreasing, the most critical management issue facing the distribution industry today is the need to efficiently organize a limited number of staff and implement delivery tasks. Conventional staffing optimization methods based on traditional organizational protocols are no longer adequate in an environment where it is necessary to respond quickly to suddenly generated tasks and to fluctuations in work efficiency related to the physical condition of the staff.
This paper introduces an original solution from NEC that uses our Autonomous and Adaptive Control technology to optimally manage warehouse staff to handle shipping tasks in real time.
1.Introduction
Spurred by the growth of electronic commerce (EC) and rapidly diversifying demand, the rate of deliveries is becoming more frequent, while lot size is becoming smaller. Together, these changes are posing a major challenge to the distribution industry, which finds itself forced to cope with ever more complex delivery tasks and ever more increasing workloads. At the same, year on year declines in the Japanese working population make it more and more difficult to meet staffing needs. Finding a way to efficiently execute delivery tasks with a limited number of the staff is now the significant management issue in the distribution industry.
For these reasons, NEC focused on enhancement of efficiency in shipping-related tasks at warehouses and distribution centers. Usually, managing staff for shipping tasks is conducted based on the on-site manager’s intuition and experience. When staffing requirements don’t correlate with on-site requirements, it can lead to over- or under-staffing, which in turn can lead to idle time while staff wait for tasks or delays because not enough staff are available to handle all tasks. Ideally, to minimize downtime and delays, staff would be optimally organized according to the changing workload. This can be expected to improve the working environment and reduce overtime costs.
However, in today’s most popular staffing optimization method, the plans are drawn up based on the analysis of past data — which makes it difficult to apply to staffing for tasks that are difficult to forecast. For example, in warehouses that may be required to respond to sudden and unexpected orders to ship stock on the same day, there will inevitably be cases where a detailed demand forecast is simply impossible. Besides, on-site workers are not necessarily capable of exerting maximum task efficiency exactly as planned every day due to unexpected days off or sickness. When all of this is taken into consideration, it becomes clear that basing a staffing plan on past tendencies is intrinsically limited. Instead, the most effective solution would be to build a mechanism to optimally adapt staffing to suit the needs of an environment that is in constant flux.
In recent years, the rapid proliferation of wireless networks and improvements in computing processing capabilities have brought into being what is now called the Internet of Things (IoT). In this environment, it is now possible to collect a variety of real-time data in the real world and instantly analyze and apply that data to real-world applications. An active leader in this field, NEC has been conducting R&D into Autonomous and Adaptive Control technology which uses data obtained from the IoT environment to control actions in the real world in order to optimize local operating conditions. By applying this technology to warehouse staffing and shipping requirements, we can help improve the efficiency of distribution operations (Fig. 1).
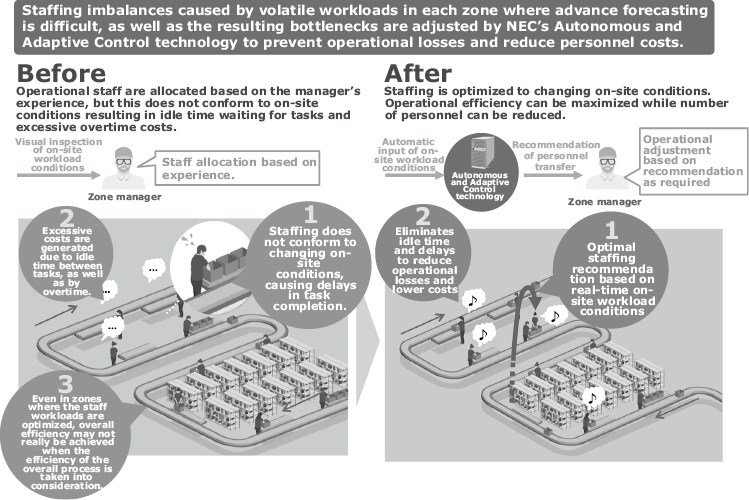
Fig. 1 Overview of warehouse staffing optimization using Autonomous and Adaptive Control technology.
In this paper, we will discuss the features of NEC’s Autonomous and Adaptive Control technology, its application to staffing at distribution warehouses, the results of simulation assessments, and the system configuration used when this technology is applied to the real world.
2.Features of the Autonomous and Adaptive Control Technology
NEC’s Autonomous and Adaptive Control technology is an original technology inspired by the mechanisms of organisms that naturally adapt themselves to changes in their environments. For instance, even without a brain, amoebas are able to catch their prey optimizing their overall shape while the nuclei inside their cells affect the adjacent nuclei mutually in order to adapt themselves to the distribution of food in the surrounding environment. The Autonomous and Adaptive Control technology is a control method that helps achieve overall optimization by dividing the overall system into discrete subsystems that determine their own behaviors both dynamically and autonomously while communicating with other subsystems (Fig. 2).

Fig. 2 Operation overview of Autonomous and Adaptive Control technology.
The main features of the Autonomous and Adaptive Control technology include the following two:
- (1)Ease of introduction
Because conventional staffing optimization control methods depend on analysis of past tendencies, they require a vast amount of past data. Moreover, that data has to be analyzed by experts who derive the rules based on the data. Introduction of such a system takes a significant amount of time and effort. Furthermore, because the entire environment has to be modeled as a system, any changes in the environment will necessitate that everything be reanalyzed and the model redesigned, adding significant ongoing costs.
NEC’s Autonomous and Adaptive Control technology, on the other hand, operates with goal-based indices (efficiency, for example) for individual subsystems that compose the overall system. Once the model of an index for a specific control purpose has been designed, all you have to do when the environment changes is adjust the parameters — which means there is no necessity to extract rules in advance and redesign models based on analyses. Consequently, the Autonomous and Adaptive Control technology can be easily applied to multiple sites.
- (2)Prompt response
In a conventional control method for staffing optimization, the entire system is modeled based on the forecast. If the forecast fails, it will be necessary to start over from scratch, meaning the entire system will have to be recalculated, which obviously makes it difficult to follow situations in real time.
NEC’s Autonomous and Adaptive Control system, on the other hand, optimizes the entire system by using localized distributed computing between subsystems. Because recalculation according to environmental fluctuation is performed locally, this technology is able to respond promptly to any changes. Also, because this distributed computing is lightweight, the technology can follow environmental fluctuations by promptly computing control values based on real-time data.
3.Application of Autonomous and Adaptive Control Technology to Warehouse Staffing
In this study, our focus is on shipping operations in a warehouse that stores stock. Operation flow is composed of three main tasks: picking items according to shipment orders, product inspection, and order merging with upstream items. Each warehouse floor is divided into multiple zones according to the types of items stocked. The staff who perform order picking, order inspection, and order merging are posted in their respective zones. Containers packed according to order units for shipment go round the zones. In each zone, ordered items are placed in the container together with items from the upstream zone (Fig. 3).
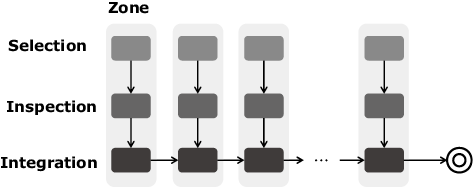
In this operation flow, if the progress of tasks in a specific zone stagnates, it will have an adverse impact on the progress of downstream processes. So it is desirable to distribute staff to ensure that progress in each zone is as uniform as possible.
When the Autonomous and Adaptive Control technology is applied to shipping operations, each zone is regarded as a subsystem. The index is set as the completion of tasks in all zones in simultaneously and staffing is controlled according to work remaining in each zone. Because any delay in a specific zone negatively impacts downstream zones, this technology evaluates the workload (remaining tasks) in each zone, while taking into account any potential impact on the overall flow. Moreover, this technology also increases and decreases the number of operation personnel based on an assessment of operational efficiency calculated based on the most recent operation results in each zone.
4.Simulation and Assessment
Assuming the shipment flow shown in Fig. 3, we performed a simulation of transfer instructions to workers using the Autonomous and Adaptive Control and determined whether or not operational efficiency would improve.
- (1)Assessment conditions
We conducted the assessment under the following conditions. We performed ten trials for each result in order to obtain a mean value that would allow us to confirm whether or not using the Autonomous and Adaptive Control would improve operational efficiency.
- Number of zones: 16
- Number of total workers: 32
- Operational efficiency in each zone: Set randomly within a range of ±30%
- Simulation duration: 7,500 seconds
- Autonomous and Adaptive Control’s computation interval of transfer instructions: 300 seconds
- Worker transfer time to adjacent zones: 5 seconds (assuming that workers do not work during the transfer)
- Order frequency rate: 35 containers every 1,000 seconds (assuming that there is no work in each zone, that the order for the first 35 containers is generated immediately after startup, and that all the orders are placed in all the zones)
- (2)System configuration
The system configuration of this simulation is shown in Fig. 4. Information on the number of workers allocated to each zone shown in Fig. 3, and operation details were created on the simulator and entered into the Autonomous and Adaptive Control system. Operation directives computed by the system were output to the simulator and applied to personnel control.
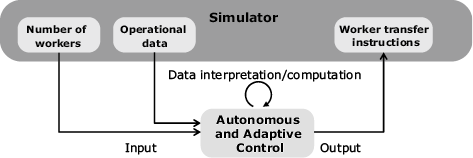
5.Simulation Results
Fig. 5 shows a chronological transition in the number of unprocessed containers in the simulation. The x-axis of the graph shows the time, while the y-axis shows the number of unprocessed containers. As soon as the simulation started, an order was placed for 35 containers. This operation continued for 1,000 seconds, resulting in a decrease in the number of unprocessed containers. Because an additional order for 35 containers was added every 1,000 seconds, the number of unprocessed containers increased steadily. When personnel was controlled by the Autonomous and Adaptive Control, the number of unprocessed containers decreased during the same period.
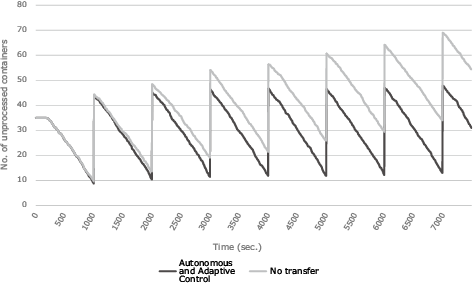
A comparison of the number containers processed during the same period confirmed that NEC’s Autonomous and Adaptive Control improved the rate by 12% over the standard operating procedure.
6.System Configuration in Real-World Applications
The system configuration when this simulation is applied to verification and operation in the real world is shown in Fig. 6. The Autonomous and Adaptive Control system is installed in a server as a software engine. To enable it to capture real-time on-site operation conditions, it is linked with a warehouse management system (WMS) and global positioning system (GPS). In addition, display monitors are installed for transmission of operational instructions from the Autonomous and Adaptive Control system to the sites.
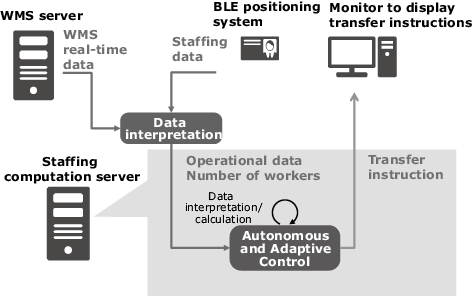
Operational data such as remaining workload and production results in each zone is derived from real-time data collected by the WMS. Remaining workload and results for each zone are calculated based on order data and operation completion data from each zone.
To track the number of the staff in each zone, workers are assigned Bluetooth Low Energy (BLE) tags which collect and pass along GPS data. As long as each worker has with them a device that allows them to be specified, we can also adopt a method that utilizes the real-time operation data provided by the device. Another possible way to collect personnel location data would be to have on-site workers and managers enter their current location into the system as and when required.
Transfer instructions from the Autonomous and Adaptive Control system can be displayed on monitors that can be viewed by on-site workers. In actual on-site applications, it would also be possible for the on-site manager to obtain the transfer instructions from the monitor and pass them on to the appropriate worker either directly or via a PA system. These are just a few of the many possible ways in which transfer instructions can be delivered to workers on-site.
7.Conclusion
With a view to helping implement more efficient shipping operation at distribution warehouses with limited personnel, we conducted simulations with the Autonomous and Adaptive Control technology aimed at improving operational warehouse staffing efficiency. Our results confirmed that this technology was capable of improving operational efficiency.
In addition to the results based on virtual data discussed in this paper, we are now implementing verification using actual data as well as conducting on-site validation tests based on real-world operations.
We believe that our Autonomous and Adaptive Control technology is ideally suited to application in a wide range of fields including energy management, taxi dispatch optimization, and IT network device optimization1). We are also refining this technology for use in location control of multiple robots.
- *Bluetooth is a trademark of Bluetooth SIG, Inc.
- *All other brand names and product names mentioned in this paper are trademarks or registered trademarks of their respective owners.
Reference
1) ICHIEN Masumi, OGAWA Masatsugu, “HEMS Data Utilization Solutions Using Autonomous Adaptive Control,” NEC Technical Journal, Vol. 10, No.2
Authors’ Profiles
KUWAKO Shizuho
Cloud Platform Division
TACHIBANA Makoto
Manager
Cloud Platform Division
OKAZAKI Takumi
Manager
Cloud Platform Division